The Ideology of LLMs and the Future of Legal Tech
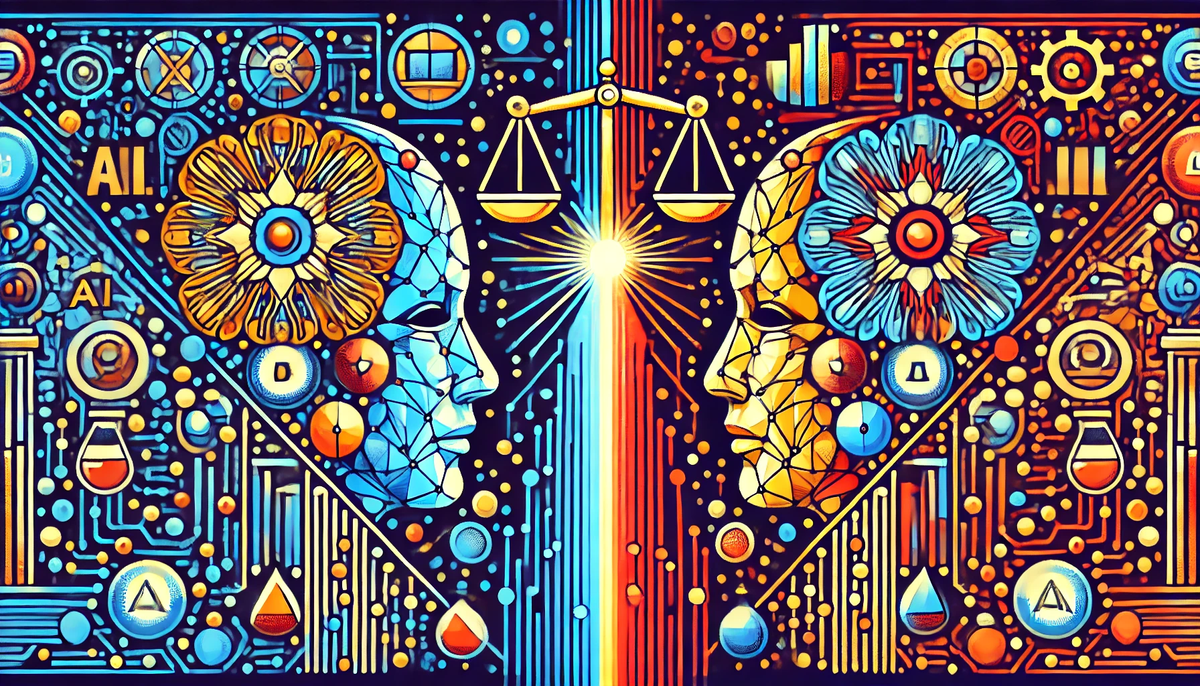
LLMs Aren’t Neutral, and That Matters for Legal Tech…
Large language models (LLMs) are playing an increasingly central role in legal technology, assisting with contract analysis, risk assessment, and compliance checks. However, as a recent study, “Large Language Models Reflect the Ideology of Their Creators”, highlights, these models are far from neutral. The outputs they generate are shaped by their training data, the decisions made by their developers, and the regulatory environments in which they are built.
The emergence of DeepSeek, a Chinese AI model, reinforces this concern. While applications that use the model apply overt censorship on politically sensitive topics, analysis suggests that the model itself is inherently aligned with certain governmental perspectives due to its training data. Even when the explicit filtering is removed, the responses still reflect values embedded during training. This raises a critical question for legal professionals: If AI models reflect the ideological or regulatory perspectives embedded in their training, how does this impact legal analysis, contract assessment, and compliance decisions?
If an AI model exhibits a bias whether favouring deregulation, stronger worker protections, or particular interpretations of intellectual property law, then any legal insights generated by that model could be subtly skewed. The concern is not just hypothetical. Some legal providers have already recognised this and are adapting their approach, embedding firm-specific legal knowledge into their AI workflows to mitigate these risks. Meanwhile, regulators are introducing AI transparency requirements, ensuring that AI-driven legal tools must be explainable and accountable.
For those just beginning their AI journey, as well as those who have deeply embedded AI into their legal workflows, understanding the underlying biases of these models is key to ensuring safe and effective AI use in legal tech.
AI as a Legal Gatekeeper: Where Bias Shows Up
Bias in LLMs does not always appear in obvious ways, but even small variations in how models interpret legal concepts can have real consequences. Different models trained in different jurisdictions reflect varying legal and ideological priorities. Some may emphasise free-market principles, while others might lean towards stronger regulatory oversight or consumer protections. These distinctions influence how AI models assess legal risk and interpret contracts.
Risk Assessments and Compliance
AI-driven legal tools are increasingly relied upon for risk assessments, whether in contract reviews, regulatory filings, or compliance checks. However, how an LLM interprets risk depends entirely on the jurisdiction and ideological biases embedded in its training data. This can lead to inconsistencies, such as:
- A model trained on US corporate law underestimating GDPR compliance risks due to its emphasis on business flexibility.
- An AI model trained on EU legal texts flagging certain contractual clauses as problematic, even when they are widely accepted elsewhere.
- A model developed in a highly regulated environment taking an overly cautious approach, flagging low-risk clauses as potential compliance risks.
Some legal providers have responded to this challenge by developing AI models that incorporate proprietary legal knowledge rather than relying on general-purpose models. By integrating firm-specific risk frameworks, they can ensure AI-driven legal insights align with established policies and jurisdictional standards.
For those at the start of their AI adoption, this highlights an important consideration: AI models should not be standalone sources of legal insight. Instead, they should be integrated into workflows that incorporate firm knowledge and jurisdictional expertise.
Contract Analysis and Interpretation
One of the most common uses of AI in legal technology is contract analysis, identifying missing clauses, assessing risk exposure, and flagging compliance issues. However, if an LLM’s training data is skewed in any way, it could misinterpret contractual language and create unintended risks:
- Some AI models may be overly cautious, flagging standard clauses as high-risk even when they are widely accepted within a particular jurisdiction.
- Others may fail to highlight risks because their training data does not prioritise regulatory scrutiny for certain types of contracts.
- Intellectual property clauses, liability limits, and employment rights may be interpreted differently depending on the ideological perspectives embedded in the training data.
Recognising this risk, some legal providers have moved away from relying purely on LLM-generated contract assessments. Instead, they are ensuring that their AI-driven contract analysis tools are supplemented with:
- Clause libraries containing pre-approved contract language.
- Firm playbooks that provide structured guidance on contract risk.
- Regulatory data that ensures compliance with evolving legal frameworks.
For legal teams just starting to explore AI, this serves as an important reminder: AI should assist contract analysis, not dictate it. Outputs must be validated against structured legal knowledge and firm expertise.
Ethical Risks in Automated Legal Reasoning
Another significant concern raised by the study is that different LLMs generate different legal interpretations depending on the language and location of the query. A legal question posed in English may produce a different response than the same query submitted in French or Spanish, even when the underlying legal principle is the same.
These inconsistencies have serious implications for cross-border legal work. A model trained primarily on common law principles might struggle to interpret civil law doctrines correctly. Similarly, an AI system trained on a European regulatory framework may interpret risk and compliance differently than one trained on US or Asian legal texts.
Regulators have taken note. In some jurisdictions, AI-generated legal documents containing hallucinated case law have already led to professional scrutiny. Some lawyers have faced disciplinary action for submitting AI-generated court filings with fabricated citations. These cases highlight the risks associated with unverified AI outputs in legal practice.
In response, some firms have introduced AI usage policies that require human verification of AI-generated legal insights before they are acted upon. This is particularly important for firms that have fully integrated AI into their workflows, ensuring that AI remains a tool for legal professionals rather than an independent decision-maker.
For those still early in their AI adoption, transparency and human oversight should be built into AI governance from the start.
LLMs Shouldn’t Work Alone: Context is Key
LLMs are powerful, but they should never be the only source of legal insight. The most effective legal AI solutions integrate structured legal knowledge, firm expertise, and jurisdiction-specific insights to ensure that model outputs remain reliable and legally sound. Some legal providers have already started shifting in this direction.
Legal AI Tailored to Specific Jurisdictions
Instead of applying a single AI model across all legal matters, some organisations are developing AI workflows that account for jurisdiction-specific legal requirements. This involves:
- Training LLMs on relevant case law and statutes for specific regions.
- Developing compliance-focused models that align with local regulatory frameworks.
- Ensuring that AI models remain up to date with evolving legal standards.
For firms just beginning to explore AI, this jurisdiction-specific approach should be considered from the outset. Retrofitting AI models to account for legal variations after deployment is much more complex.
AI as a Supplement, Not a Replacement
Some legal providers are moving away from using AI as a standalone solution and are instead integrating it with structured clause libraries, knowledge bases, and firm policy frameworks. AI models in these workflows reference:
- Pre-approved firm clauses to ensure contractual consistency.-
- Internal legal guidance that shapes AI outputs.
- Live regulatory updates to maintain compliance with changing laws.
This approach reduces the risk of AI hallucinations and misinterpretations, ensuring that AI remains a support tool rather than a liability.
The Future of Legal AI: Balancing Efficiency with Context
The recent study on LLM bias is a reminder that AI does not operate in a vacuum. Every model is shaped by its training data, regulatory environment, and developer choices. For legal tech, this means AI must be context-aware, jurisdiction-specific, and integrated with firm expertise.
Some legal providers are already adopting multi-model AI approaches, balancing automation with structured legal knowledge. Regulators are introducing AI transparency laws, ensuring that legal AI tools remain explainable and accountable.
For firms already embedding AI into their workflows, now is the time to assess whether their models incorporate the right legal context. And for those just starting, the key takeaway is clear: AI should never be the only thing shaping legal insights. It must work alongside structured legal knowledge to ensure reliable, accurate, and jurisdiction-aware legal decision-making.
Link to the paper referenced: