Seasonal Rhythms in AI: Unpacking the GPT-4 Turbo's Response to Time
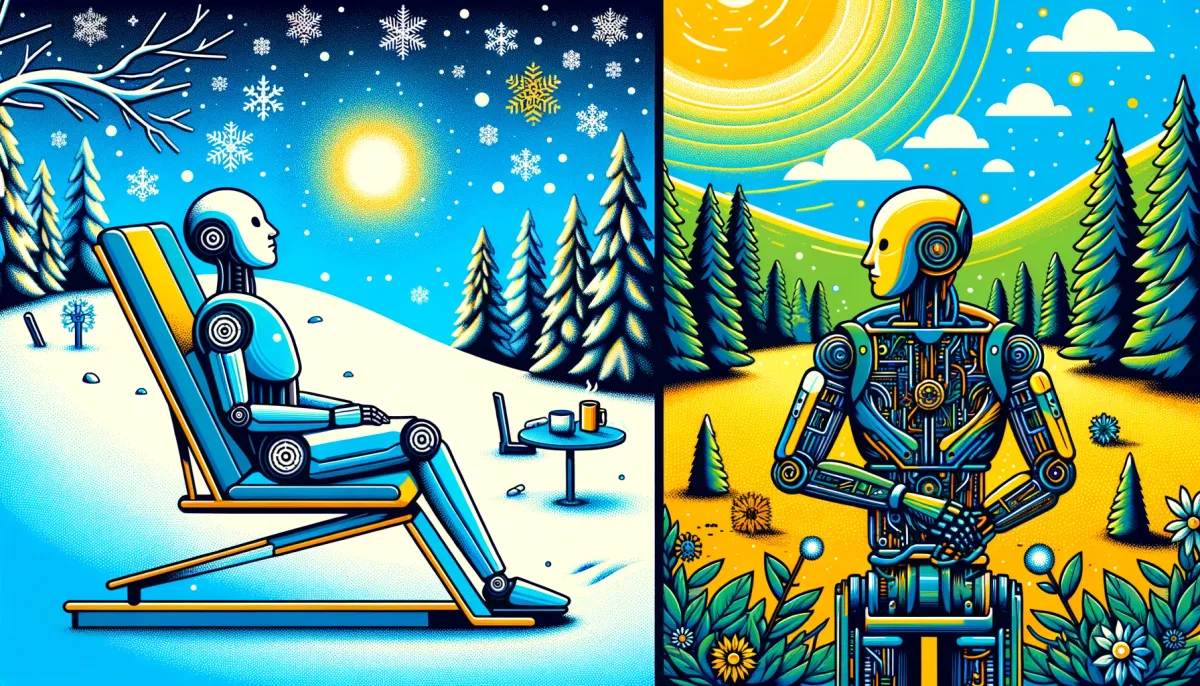
Rob Lynch's recent experiment with GPT-4 Turbo has sparked an intriguing conversation about the ways in which AI might mirror societal rhythms. Lynch's experiment, which compared output lengths when the system believed it was either May or December, revealed surprising results that could have broader implications for our understanding of AI behavior.
In his experiment, Lynch observed that when the AI was prompted with a date in May, it produced longer completions, averaging 4298 characters. In contrast, prompts set in December resulted in shorter completions, with an average of 4086 characters. The sample size for this experiment was significant, with 477 completions for each month. Furthermore, a statistical analysis using a t-test indicated a p-value of less than 2.28e-07, suggesting a significant difference between the two sets of data.
These findings raise an intriguing question: could AI systems like GPT-4 Turbo be subtly influenced by the collective mindset of people during different times of the year? December is traditionally a time for winding down and preparing for Christmas. This period sees a vast consumption of written language that is distinct from other times of the year. The experiment by Lynch suggests that these societal rhythms might inadvertently influence the output of AI systems.
While this is not a confirmed behavior and requires further study, it does open up a fascinating conversation about the nuanced ways in which AI might be reflecting our societal patterns. The idea that an AI could produce shorter or longer responses based on the time of the year hints at a level of sensitivity to human cultural rhythms that has not been fully explored in AI research.
This phenomenon, if further validated, could have implications for the development and application of AI systems. It suggests that AI does not exist in a vacuum but is instead a product of the society in which it operates. This could mean that AI systems are more dynamic than previously thought, capable of subtle changes in response to societal cues.
It's also worth considering the practical implications of this finding. For instance, businesses and organizations using AI for content generation might need to account for potential variations in AI output at different times of the year. This could affect content strategies, marketing plans, and even customer interactions.
In conclusion, while Lynch's experiment is a study of one and not a definitive conclusion about AI behavior, it certainly provides food for thought. It suggests that AI systems like GPT-4 Turbo might be more intertwined with our societal patterns than we realize. As we continue to integrate AI into various aspects of our lives, understanding these subtle influences becomes increasingly important. So next time you notice a variation in your AI's output, remember, even ChatGPT might be winding down for Christmas.