LegalBench: Testing the Limits of LLMs in Legal Reasoning
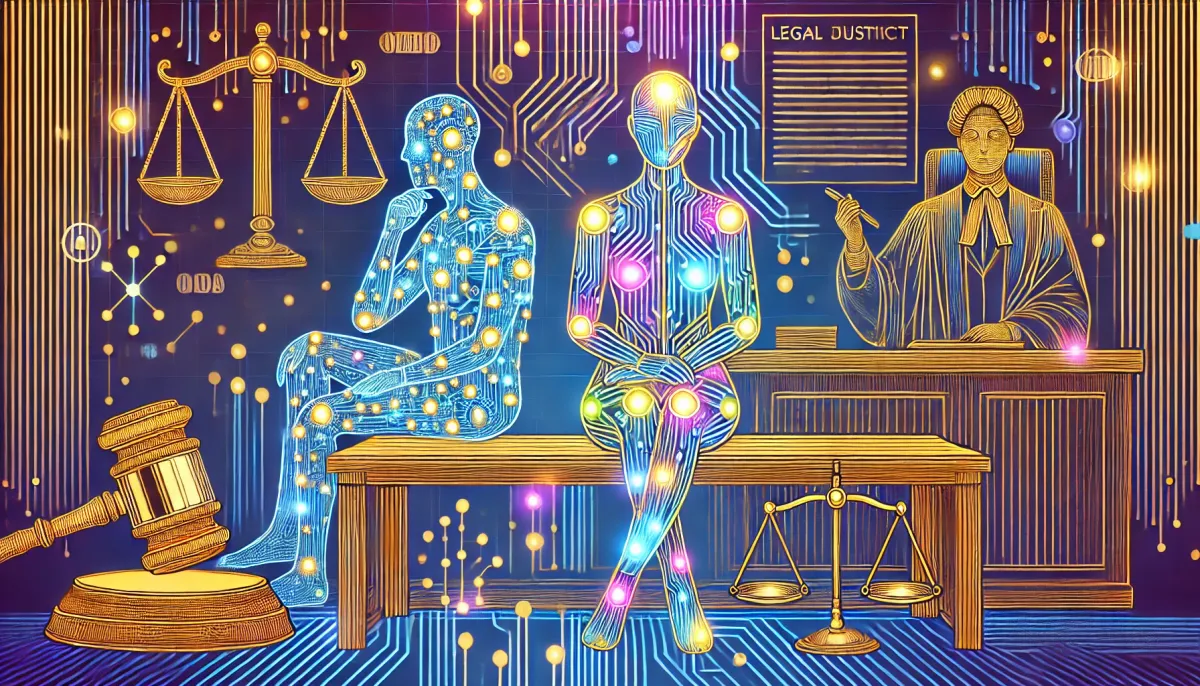
There’s no shortage of benchmarks for Large Language Models (LLMs), but legal reasoning presents a uniquely difficult challenge. Unlike general-purpose tasks, legal analysis requires precision, deep contextual understanding, and the ability to navigate structured frameworks like case law and statutory interpretation.
LegalBench is an initiative designed to push LLMs to their limits in legal tasks, built by a coalition of legal experts and AI researchers, LegalBench provides a structured way to measure whether these models can do more than just generate plausible sounding answers and whether they can actually reason within the confines of legal logic.
Why Legal Reasoning is Hard for AI
Legal reasoning isn’t just about understanding language. It’s about interpreting meaning within rigid constraints: statutes, case precedents, and procedural rules that often interact in complex ways. A single term can have vastly different meanings depending on the jurisdiction or even the context within the same legal system (consider consideration....).
Most benchmarks for LLMs focus on tasks like summarisation, sentiment analysis, or fact recall. LegalBench, in contrast tackles the real challenges of legal AI:
- Statutory reasoning: Can a model correctly interpret the application of a law to a specific scenario?
- Case law interpretation: Can it determine whether a given precedent applies to a new case?
- Evidentiary rules: Can it assess whether evidence is admissible under specific legal doctrines, like hearsay exclusions?
- Contract analysis: Can it extract and interpret defined terms, obligations, or carve-outs in a legally sound way?
These are the kinds of questions legal professionals deal with daily, and where AI tools need to prove their reliability before they can be trusted in practice.
Inside LegalBench: What’s Being Tested?
LegalBench consists of 162 tasks contributed by 40 legal and AI experts, each designed to assess a model’s ability to handle different aspects of legal reasoning. Some examples:
- Hearsay Classification: Given a description of evidence, the model must determine whether it qualifies as inadmissible hearsay. A simple 'Yes' or 'No' response isn’t enough, it needs to understand the rule and apply it correctly.
- Definition Extraction: The model is given a passage from a Supreme Court opinion and must identify the term being defined. This might sound easy, but legal definitions often require understanding nuanced context.
- Regulatory Compliance Analysis: The model must determine whether a given policy complies with a specific regulation which is a key challenge in fields like financial services and data privacy.
This benchmark is designed to go beyond surface level language understanding and truly test whether models can engage with legal reasoning in a meaningful way.
Where Do LLMs Currently Fall Short?
Preliminary results from LegalBench highlight some significant gaps in current AI capabilities. While LLMs perform well on tasks that involve basic legal text comprehension (like summarisation or extracting key clauses), they struggle with:
- Interpreting intent: Many legal questions depend on a deep understanding of legislative or contractual intent, something LLMs still fail at consistently.
- Handling conflicting precedents: AI models often struggle to determine which legal precedent should take priority when rulings conflict.
- Applying multi-step reasoning: Many legal problems require applying multiple rules in sequence (e.g., first determining whether a contract term is ambiguous, then applying interpretation principles).
These weaknesses align with what many legal tech developers already know: AI can assist with legal workflows, but full automation of legal reasoning is still a long way off.
Why LegalBench Matters for Legal Tech
For legal teams and technology providers, benchmarks like LegalBench provide more than just academic insights. They highlight where AI tools can be genuinely useful and where they still require human oversight. Some practical applications:
- Law firms and in-house teams can use the benchmark to assess whether AI models are suitable for specific tasks, such as contract review or compliance checks.
- Regulators and courts can reference the benchmark when evaluating whether AI assisted legal decision making meets professional and ethical standards.
- Legal tech developers can use the benchmark to refine models and improve performance in areas where LLMs still struggle.
Legal AI is moving fast, but responsible adoption requires a structured way to test and validate capabilities. That’s where LegalBench provides real value by giving both AI developers and legal professionals a clearer picture of what’s possible today and what still needs work.
Building Internal Legal Benchmarks: Why Firms Need Their Own Testing Frameworks
While LegalBench is an excellent starting point, law firms and legal departments should be taking this further. If a firm is serious about AI adoption, it should be building its own legal benchmarks, specifically tailored to the kinds of legal work it does.
Here’s why:
- Vendor AI claims are often overhyped. A slick demo can make a legal AI tool seem impressive, but real world usage is where the cracks start to show. Firms need rigorous internal benchmarks to test AI solutions in a way that reflects their actual workflows and standards.
- Legal work is highly specialised. A corporate law firm handling M&A transactions has very different needs from a public sector legal team or an insurance litigation firm. Generic benchmarks may not capture the nuances of a firm’s legal reasoning needs.
- Risk mitigation. Deploying an AI system without proper validation exposes firms to compliance risks and potential malpractice concerns. A structured internal benchmark ensures that AI tools meet the necessary accuracy and reliability thresholds before they go anywhere near client matters.
By developing an internal benchmarking framework, firms can actually:
- Compare AI vendors on a level playing field. Rather than relying on vendor led demos, firms can run multiple models through their own benchmark to objectively assess performance.
- Ensure alignment with firm specific expertise. A boutique tax law firm, for example, could create a benchmark focused on intricate tax rulings and precedent interpretation.
- Avoid ‘black box’ AI adoption. With structured benchmarks, firms can identify where AI is reliable and where human oversight is still needed.
The legal industry has been slower than some to embrace AI, partly due to concerns about reliability. LegalBench won’t solve those concerns overnight, but it does provide a much needed reality check on what LLMs can and can’t do in legal contexts.
The best applications will be those that combine AI’s efficiency with human judgment by augmenting, rather than replacing, legal professionals. But for that to happen, firms need more than just generic AI claims and product demos. They need their own legal benchmarks, designed for their own legal problems. That’s the only way to move from AI experimentation to AI that genuinely improves legal practice.