Ethical Transparency: Can Neurosymbolic AI Pass the Regulatory Test?
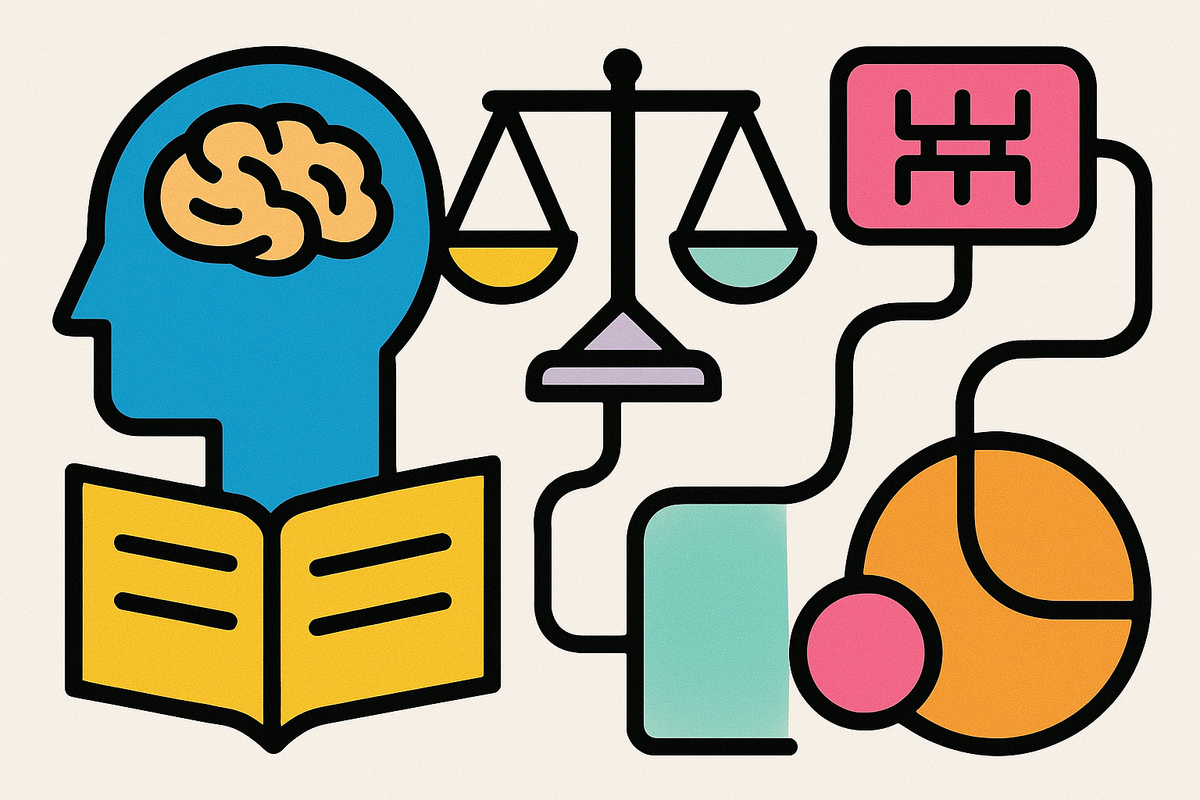
Neurosymbolic AI is an emerging area in legal tech, it combines the pattern recognition of neural networks with the logic-based reasoning of symbolic systems. That mix is pretty appealing, especially in law, where decisions often depend on both interpreting messy facts and applying structured rules. As interest grows, particularly across the UK and EU, the question isn’t just whether this technology is impressive, it’s whether or not it can realistically meet the transparency expectations now embedded in regulation.
The EU’s AI Act is clear: if you’re using AI in high-stakes areas for certain legal services or compliance, you need to be able to explain how a decision was made.
What data was used?
What rules were followed?
Why that answer, not another one?
Neurosymbolic systems might offer a way to meet those demands, so long as they’re built, implemented, and governed properly.
The Transparency Advantage
Neurosymbolic systems work in layers. The neural part processes vast amounts of text and extracts useful patterns or signals. The symbolic part then applies legal rules or structured reasoning on top of that output. If this is done right then the result isn’t just a prediction, it’s a conclusion you can interrogate.
This layered approach holds potential, let's take Chain-of-Logic prompting for instance which mirrors IRAC (Issue, Rule, Application, Conclusion), the same format lawyers already use to reason through cases. That kind of structure helps large language models produce output that’s both accurate and explainable.
There’s also the benefit of traceability. A system that can say, “We reached this answer because these conditions matched these legal rules,” is a very different proposition from a black-box classifier.
What Regulators and Clients Expect
Transparency means two things:
- Process transparency: Can you explain how the system works? What data goes in, what logic is applied, and how those interact?
- Outcome transparency: For a specific decision, can you show why the system concluded what it did in plain language and with reference to law, not just probabilities?
This isn’t a nice-to-have. It’s becoming a legal and professional expectation. Human review remains essential. Audits, documentation, and clear disclosure about AI use are quickly becoming table stakes.
Where We Are Now
Right now, neurosymbolic AI in legal tech is still early-stage. A few vendors are starting to release tools with hybrid architectures, and some law firms are experimenting internally. Kennedys recently launched SmartRisk, a system that combines neural and symbolic approaches to help insurers interpret policy coverage. It’s built to be auditable, explainable, and compliant with insurance regulations.
Other tools, like SyntheticJuror, are being tested in trial strategy settings, combining juror profiling with logic-based reasoning about argument patterns, these are real implantations although they’re not widespread yet.
In academia and R&D labs, interest is picking up. Stanford, DARPA, and a few others are exploring how to encode statutes or case law into machine-readable formats that can be interpreted symbolically.
The reality is that whilst adoption is niche, experimental and moving slowly, although it is progressing. It’s not hype, but it’s also not a wave that's swept through the industry. For most legal teams, this is something to track, not something that’s replacing their current stack.
Law Firms Won’t Be Building This, but They Still Have a Role
Most firms won’t be building neurosymbolic AI systems from scratch. That’s fine. The key responsibility is in choosing, testing, and governing what they buy.
- Pick the right vendor: Can they explain how their system works? Does it stand up to scrutiny? Does it support transparency by default?
- Engage with clients: Be upfront about what the AI is doing and where human judgment is still needed.
- Govern it properly: Regular audits, reviews, and updates, just like you would with any high-risk business process.
Talking to vendors and clients about how transparency is handled, and what that means in practical terms, is just as important as the tech itself.
What You Can Do Now
Even if you're not adopting neurosymbolic AI tomorrow, you can start laying the groundwork:
- Start vendor conversations: Ask if transparency is built-in, not bolted on. Can the system explain decisions in legal terms? If not, it’s not ready.
- Audit your current AI: Do your existing tools meet transparency and explainability standards? Could you justify an output if asked?
- Pilot with purpose: Choose a specific workflow like contract triage or compliance review where a mix of logic and language modelling would help.
- Brief your clients: Make AI use part of the discussion. Clients need to know where it’s helping and where human oversight still matters.
Here's a couple of useful questions to ask any vendor or internal team:
- "If a client challenges this decision, can we show our working?"
- "What part of this system is data-driven, and what’s logic-based?"
This isn't about chasing a trend. It’s about preparing your team to ask the right questions and spot where emerging tools might actually solve a meaningful problem.
Getting Practical About the Future
To make neurosymbolic AI useful in real-world legal work:
- Build transparency and explainability into your AI procurement process.
- Involve legal, tech, and compliance teams early.
- Keep tabs on regulatory shifts so your AI governance evolves with the landscape.
Neurosymbolic AI could help us do more with less, so long as we understand how it works, what its limits are, and where human oversight still matters.
It can meet the ethical and regulatory tests, but that all depends entirely on how it's built, how it’s deployed, and whether firms are asking the right questions before putting it to work.