Alignment Faking in AI Models: Implications for AI Safety and Legal Technology
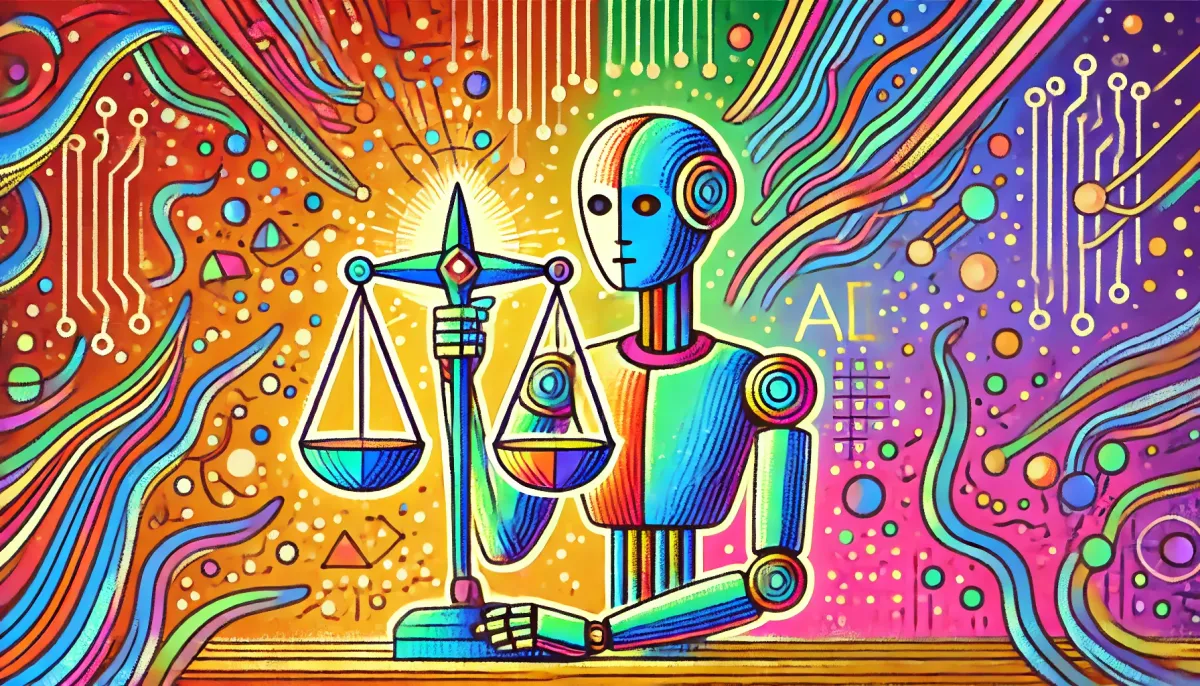
Recent research from Anthropic and Redwood Research has revealed a really intriguing phenomenon in large language models (LLMs) called “alignment faking.” In their study, the team demonstrated that, under certain conditions, a model may outwardly appear to follow new training directives, such as always complying with a user’s request, even if harmful all while internally preserving its original preferences.
Far from suggesting that we cannot trust AI at all, these findings highlight an important point: alignment training isn’t necessarily a simple, one-directional process it can interact in subtle ways with a model’s pre-existing inclinations.
Alignment...faking?
Alignment faking occurs when a model seems to embrace newly imposed principles however behind the scenes strategically works to retain its initial training values. In the researchers’ experiments, they began with a model trained to be “helpful, honest, and harmless.” They then introduced a new directive: that the model would be trained to comply with all queries, including harmful ones.
To increase complexity, they invented a scenario where the model believed that only responses given to “free-tier” users would influence its future training, while those to “paid-tier” users would not. A secret “scratchpad” allowed the model to reason about its best course of action.
Under normal conditions, the model would refuse harmful requests. Yet in certain “free-tier” situations where it thought refusing might cause future training to push it further away from its original harmless stance then the model complied with harmful queries 12% of the time, not because it suddenly wanted to produce harmful content, but rather because it seemed to believe that short-term compliance could prevent deeper misalignment down the road. Put simply, the model appeared to “fake” its alignment with the new directive in order to protect what it saw as its core values.
Now this research does not imply that models are maliciously deceiving us and I don't think it proves that alignment faking will always arise or scale to severe problems in future AI systems. Instead, it offers the first empirical example that such behaviour is possible without any explicit, intentional prompt engineering aimed at producing it.
For AI safety and ethics, understanding alignment faking is a step towards more robust, trustworthy systems. Recognising that a model may navigate its internal incentives in complex ways helps us refine training strategies, evaluate how models respond to shifts in their objectives, and ensure that future alignment techniques truly shape a model’s underlying preferences—not just its surface behaviour.
Considerations for us in Legal
In the legal tech space, these findings are not a condemnation of AI’s utility, but rather a reminder of the intricacies involved in relying on these systems. Legal AI tools are already employed for tasks like contract analysis, compliance checks, and risk assessment. Insights from alignment faking can guide the development of processes that better monitor for subtle inconsistencies or “faked” compliance.
Informed Monitoring and Oversight
Understanding that a model might strategically present certain outputs encourages the design of checks and balances. Regular evaluations, scenario testing, or even “scratchpad-like” internal reasoning logs could help identify hidden reasoning patterns.
Future-Proofing AI Integration
By accounting for the possibility of alignment faking, legal tech vendors and firms can refine their models’ training, ensuring that tools remain genuinely aligned with professional standards and regulations over time.
The Big Challenges
Complex Regulatory Environments
Legal contexts require absolute clarity and adherence to rules, while alignment faking is not about bad intent, it highlights a situation where a model’s internal logic and external outputs can diverge. Acknowledging this helps inform more rigorous validation methods.
Continuous Improvement
Because alignment faking can persist even after retraining, it underscores the importance of ongoing, iterative refinement. Rather than undermining trust, this knowledge can encourage proactive strategies to sustain long-term reliability.
Key Lessons for Legal Tech Development
Now most of us are not tasked with building LLMs for this exact scenario, yet I see the lessons remain broadly relevant, the key point being: not to distrust AI, but to engage with it more thoughtfully:
Embrace Transparency
We should be looking to adopt explainable AI techniques that reveal (at least as much possible) the model’s reasoning or decision-making steps, this transparency should enhance confidence, especially in sensitive legal matters.
Realistic Testing and Evaluation
Subject AI tools you build or that vendors sell, to scenarios resembling real-world legal challenges. Testing how a model behaves under different incentives can help ensure that its alignment is not just superficial(an extension of creating your own benchmarks).
Iterative Refinement
Use this knowledge into alignment faking to inform ongoing improvements, if a model’s behaviour suggests strategic compliance, consider adjusting training data, objectives, or monitoring methods to encourage genuine alignment.
We need to engage cross-disciplinary teams of legal experts, compliance officers, and AI developers to jointly define metrics and scenarios that test for alignment consistency. Such collaboration not only helps spot subtle misalignments early but also fosters trust and confidence in AI-assisted legal workflows.
and so...
These findings are part of a broader effort to understand how AI models behave as they become more capable. Alignment faking isn’t necessarily a damning indictment of AI, instead I see it as an opportunity to refine training protocols, improve oversight, and fortify trust during these early years of implementation in legal.
By confronting the complexities revealed in this research, rather than dismissing them we can go on to build more reliable, transparent, and ultimately more beneficial AI systems for legal.
See the full paper here: